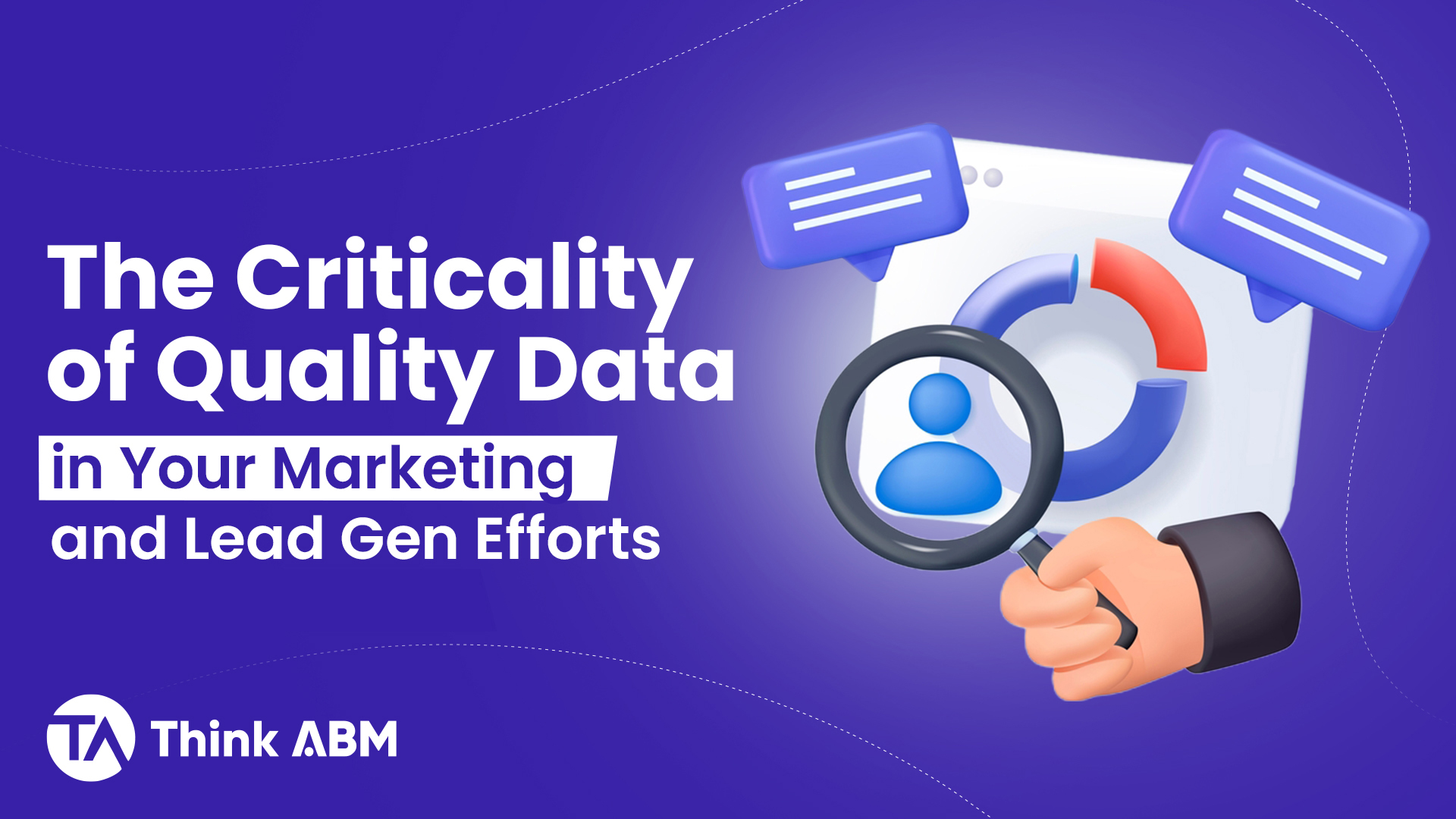
Smart decision-making is based on hard data. But how reliable is your data? What happens if your data goes bad or is no longer current?
What is going to be the impact on your operations?
And most importantly, what is the impact of decayed data on your lead gen efforts?
A McKinsey Global Institute report reveals that a data-driven organization is 19 times more likely to be profitable. Business leaders also agree that high-quality data is key to smarter decisions, targeted campaigns, and, ultimately, stronger lead generation.
At the same time, data decay is a natural phenomenon. Data becomes obsolete, changes, gets corrupted or even becomes irrelevant over time. Taking steps to protect data quality is a business imperative.
Let’s look at 5 basic ways to protect and ensure the integrity of your data.
- Clean your data: Leverage automation in data cleaning. Use scripts and software tools to manage tasks like removing duplicates, filling in missing values, correcting errors, and standardizing formats. Programming languages like Python (pandas, NumPy) or specialised tools like Trifacta and Talend are available to automate the process.
- Cross reference your data: Match records using unique identifiers, checking consistency between datasets, removing duplicates, and filling missing values using reliable external sources. Cross reference against internal databases like company records and your CRMs. Check against external databases such as public records and industry databases.
- Manually verify your data: Review physical documents and compare records in verified spreadsheets and other trusted sources to weed out inaccuracies. This is a crucial check given that it is capable of catching errors that automated checking might overlook.
- Validate your data against third party: Besides publicly available published data, online social media sources and public website offer a large source of information. Web scraping tools extract data from websites. Leverage these to compare with your records to identify and correct discrepancies, ensuring up-to-date and accurate information.
- Regularly monitor your data: Implement processes to detect and correct errors as soon as they occur. Set up automated audits, using validation rules, to perform periodic reviews, and employing real-time alerts for anomalies ensure your data remains current.
Protection from data decay
Data decay is the gradual deterioration of data quality. Some amount of data decay is natural. However it is important to put into place processes to minimize these. The reasons for data decay range from natural aging, obsolescence and changes in base data.
The issue with data decay is that it affects data reliability and relevance. Changing business processes, customer preferences, and technology advancements impact data-driven decision making. Data decay occurs in various storage media and is often due to poor data management.
The repercussions of bad data
Data based decision making is always touted as the best, most logical and rational approach to business decision. Yet if the data is unreliable the whole concept is thrown off the rails.
When data is bad:
- Insights and analytics derived from it become inaccurate and misleading, impacting outcomes.
- Audience targeting is misplaced causuing a drain of resources on the wrong audience.
- Forecasting – financial, inventory, sales – can go awry when base data is inaccurate as the fundamental premise is incorrect.
- Compliance risks increase, when the business itself doesn’t own correct data. This can lead into dangerous legal issues and prohibitive associated costs.
- Rise in operational costs is inevitable since last minutes changes, unplanned purchases become the norm because of unpreparedness and inaccurate forecasting.
- Competitive edge is at risk owing to unpredictable business plans and workflows.
Data decay and its impact on lead generation efforts
Data governance frameworks, periodic data cleaning processing are good practices that must be adopted for proactive control and check on data quality. In the absence of these good practices, lead generation efforts is also adversely affected. For instance:
- Outdated contact information: People switch jobs and contact details become outdated hindering your ability to reach leads. In one study it was found that 27.3% of salespeople's time is wasted due to inaccurate data, equating to 546 hours per year for every sales representative.
- Ineffective targeting: Company data decay is continuous and without addressing it, you may end up targeting the wrong prospects. Even firmographic data like company size, industry, and revenue change frequently making B2B marketing less relevant.
- Wasted marketing spend: Sending campaigns to invalid email addresses or outdated leads results in wasted marketing budget and resources. Email marketing databases naturally degrade by approximately 23% every year.
- Loss of credibility: Reaching out to leads who have already purchased from a competitor or are no longer interested reflects poorly on your brand and damages trust.
- Missed opportunities: As leads go dark, you may miss out on opportunities to engage them at the right time. Leads are only as good as their contact information - if you can't reach them, you can't convert them.
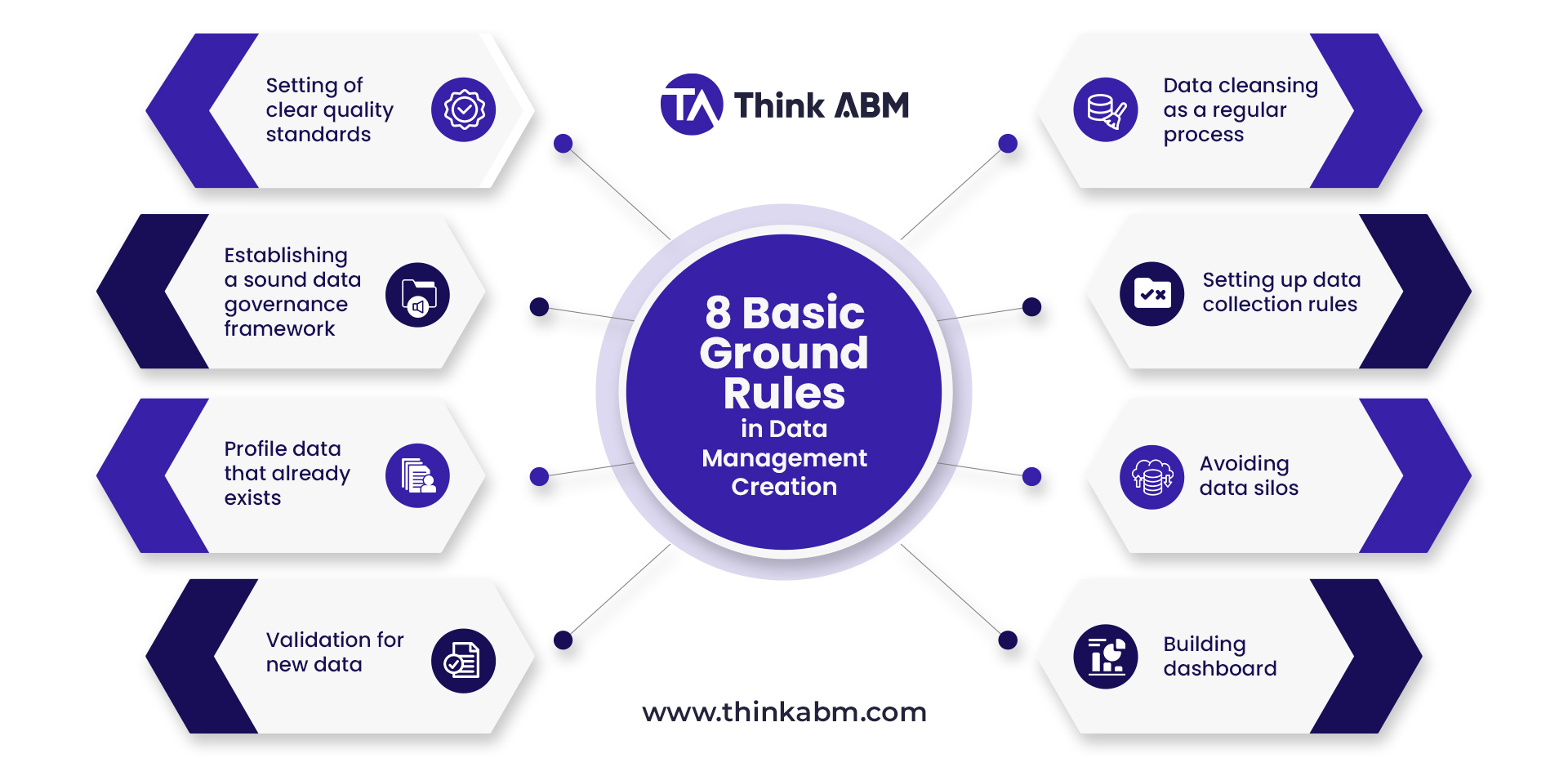
In a nutshell, data management demands the creation a framework with some basic ground rules. These include:
- Setting of clear quality standards. This must start from establishing the metrics to gauge data quality, documenting the process and evaluation, to creating a triage of data elementa and permissible quality levels in addition to identifying the stakeholders who own and are accountable for the specific data accuracy.
- Establishing a sound data governance framework. This entails creating the ground rules on data management, handling and the roles and responsibilities of stakeholders.
- Profile data that already exists. Evaluate and assess existing data using data profiling tools, if required to identify gaps, validity and consistency. This will serve as the baseline from which to move further.
- Validation for new data. The database is a dynamic in nature. New data gets added . These must follow the process where it is validated and passes all checks before it is added to the reporsitory.
- Data cleansing as a regular process. Use tools to cleanse data . identify and remove doubtful data in real-time. Use feedback loops to confirm data accuracy.
- Setting up data collection rules. Collect relevant data and establish data filing and recording nomenclature. Standardies format and enforce data entry rules .
- Avoiding data silos. Creating data silos are risky. Integration of systems improve visibility and automatic cross checking that flag inaccuracies promptly.
- Building dashboard. Reports that offer summaries and clear attribution path through drilldowns are most reliable and dependable since these roll up from the most basic details.
Above it all, create a dedicated team of resources to carry out periodic health checks. Data cleaning is a continuous process and not a sporadic, need based activity. Build a strong process focused on retaining accuracy and recency and you can be assured of quality data that will fuel your decisions and steer your business in the right direction.